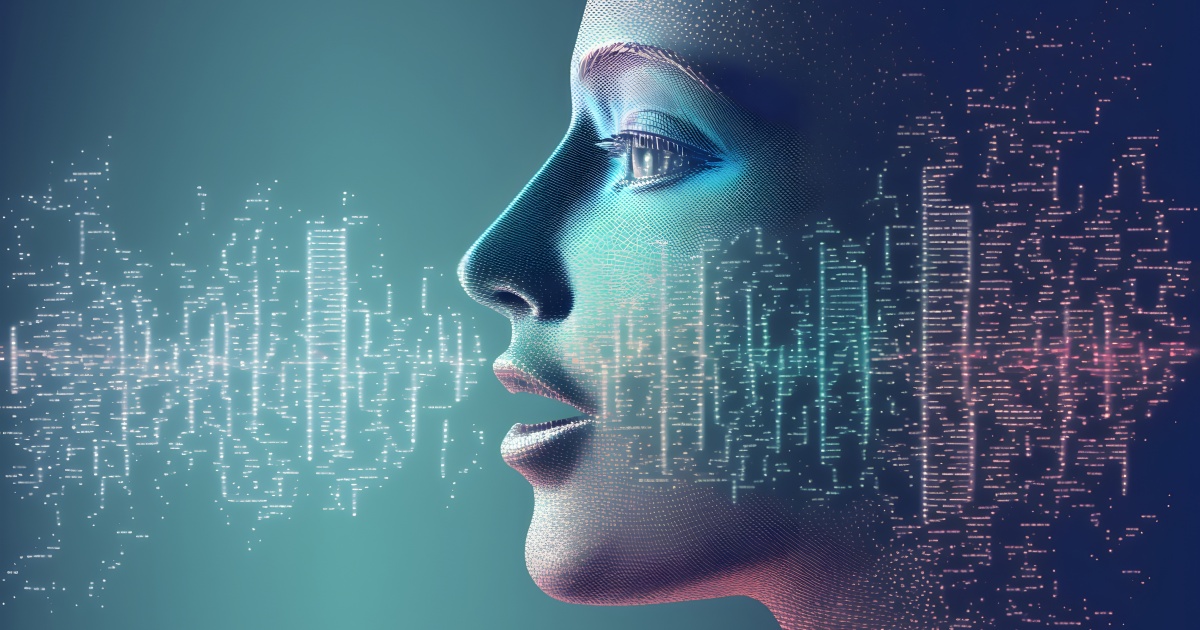
In the second part of this series,we move beyond explaining how business users must drive AI implementations and explores how IT can help them utilize AI to deliver better business outcomes as an example of effective collaboration. If you missed Part 1, you may find it here.
Organizations have long attempted to reduce friction in customer experience by deploying the latest technology. The trouble is that these efforts often fall short due to limitations in the technologies’ capabilities. For example, the newest chatbots and intelligent virtual agents (IVAs) have not solved the most important issue: finding the correct answers to commonly asked questions from customers. This is because they don’t address the core underlying search problem, which is the challenge of searching unstructured, or free-form, text. Keyword-based search is sufficient in answering only a portion of call center queries due to this lack of ability. A telling metric is that 29 percent of customers drop from the queue completely when a brand doesn't respond to their questions instantly.
On a related front, most artificial intelligence-related development initiatives fail – around 90 percent – due largely to communication and process problems between AI teams that develop and train the models for their applications and the business users who will utilize them daily. Going back to the previous example, the issues underlying the poor search outcomes must be resolved before companies can successfully serve their customers and employees, providing a consistent and positive user experience across all channels.
Organizations are now finding it possible to connect search and AI in an effective way by applying solutions such as natural language processing (NLP) to provide the previously missing link. With NLP, IT can address and solve the underlying search problem these companies always have wanted to resolve: allowing users to quickly and easily find answers in unstructured text using natural language, without the need for human intervention or multiple tiers of support.
Natural Language Search (NLS) as the Missing Link
Leveraging NLP, natural language search enables individuals to phrase questions using their own words – as if they were speaking to a person – and instantly receive all the relevant answers back to them. When a customer or a support agent has a question about a specific product or service, they can type the question into a search bar or a chatbot and receive a list of results that point to the exact sentences and passages containing the answers. This means they no longer need to sift through a results list or an entire document to find the relevant information. Instead, the solution returns results similar to having a designated expert customer support representative or personal assistant standing by to point them to the precise information needed.
NLP technology has improved rapidly in the last few years such that search engines can now understand natural language questions, meaning users no longer need to know complex search syntax. These advanced search tools also possess the ability to detect the same question phrased in different ways and return the right answers, regardless of how the question is asked. This modern user-centric design approach to search enables a number of benefits, including more accurate results, increased user productivity, and reduced cost.
Early issues for LOB Users
There were – and still are – a few common problems that companies experienced when putting AI to work in their customer-facing apps, including:
- Lack of Understanding: Early on, many organizations failed to spend enough time fully comprehending users’ needs, which prevented them from providing the tools and experiences their customers had hoped for.
- Complexity: The second issue organizations experienced was a lack of user-friendly solutions for line-of-business (LOB) teams, which prevented them from quickly adopting and fully exploiting any of the AI applications that were delivered to them. As a result, they were forced to endure lengthy training and learn new technical skills – tasks that were often impractical, given their daily workloads.
- Misalignment: The third problem is that, during the procurement process, AI teams often select tools that are optimized to support the workflows for their own jobs rather than solutions built for LOB users. As a result, those tools were never fully adopted or used by LOB, leaving businesses unable to reap their benefits.
How Technical Managers Can Drive NLP Usage
To help ensure AI success, technical teams must fundamentally understand the big-picture viewpoints from the C-suite, the business goals and processes, and the user behaviors of LOB. It is also critical that technical teams not become distracted by shiny new technologies that are impractical to implement and nearly impossible for users to leverage. To streamline this effort, technical and AI teams should create a workflow list that accomplishes the following:
- Involves all parties by inviting business users into the conversations from the start, since developers need to understand details of the business' needs and line-of-business users' workflows, business objectives, and behaviors in order to build an AI solution that users are able to actually use day-to-day.
- Explains AI outcomes for LOB personnel (e.g., call centers) and how a specific AI project can help them achieve their goals.
- Understands how LOB will use the AI-powered results in their workflows and how IT can seamlessly build in an AI output that is integrated into business processes to drive productivity and deliver business impact.
- Monitors the speed of LOB adoption of the AI tools, develops an efficient process to incorporate their feedback when using those tools, and then continues to fine-tune or even let LOB optimize those AI solutions on their own. This is where low- or no-code development comes into play.
Whether an organization delivers an AI-as-a-service offering or uses an in-house-built solution, immersing the business users in the end-to-end AI development cycle is the key to ensuring AI success. It is a collaborative effort and may take some time to develop the rhythm with the various teams involved in the development process, but the results will be worthwhile. Bringing the relevant teams together into the AI initiatives will pave the way for enterprise-wide AI adoption and will help create tangible business benefits for your customers.
About the Author: John Reuter is the Chief Strategy Officer at Kyndi, a global provider of the Kyndi Platform for the Natural-Language-Enabled Enterprise, an AI-powered platform that empowers people to do their most meaningful work. To learn more visit https://kyndi.com/ or follow them on LinkedIn and Twitter.
Edited by
Erik Linask